VIII. Conclusion: Graph Databases as a Strategic Asset for the Future of Enterprise AI
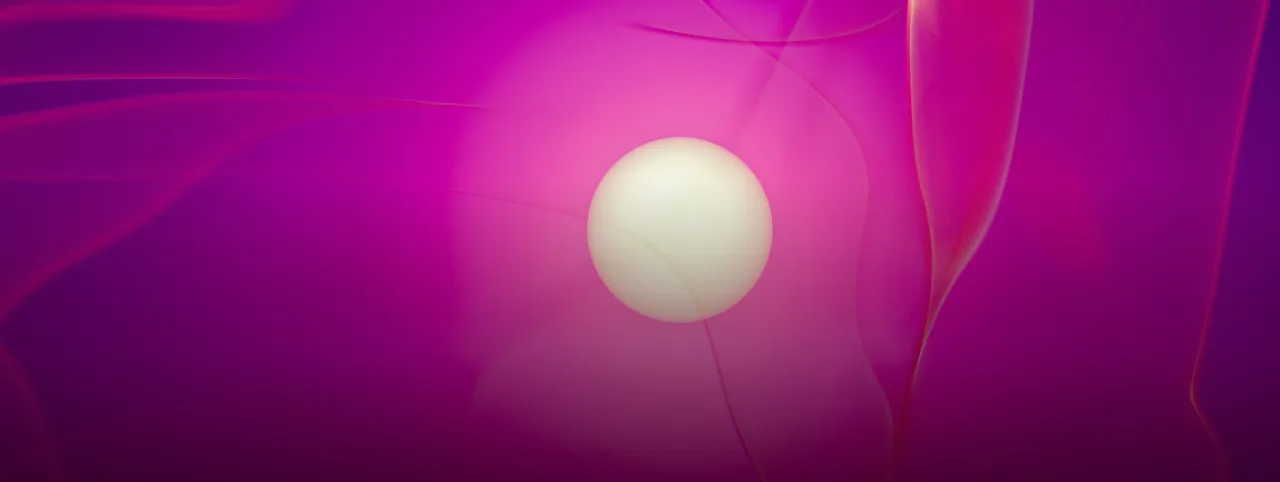
As AI and machine learning become central to enterprise innovation, graph databases emerge as a strategic asset, offering a powerful and flexible data foundation for advanced AI applications. This final section summarizes the critical benefits of graph databases, the unique role they play in supporting AI-driven transformation, and the long-term value they bring to organizations across industries, particularly in finance, healthcare, retail, and telecommunications.
1. Identifying High-Impact Use Cases for Graph Databases
Graph databases provide unparalleled capabilities for relationship mapping, contextual insights, and real-time analytics, which are essential for enterprise AI applications. Unlike traditional databases, which struggle with dynamic and interconnected data, graph databases are optimized for exploring complex relationships at scale, supporting applications that demand high levels of flexibility, speed, and insight. The key benefits include:
- Enhanced Relationship Mapping: Graph databases excel at identifying patterns and relationships within data, which is critical for understanding customer preferences, identifying fraud, and optimizing supply chains.
- Real-Time Decision-Making: By enabling real-time traversal of data relationships, graph databases empower enterprises to make faster, data-driven decisions, enhancing responsiveness in areas like customer service, compliance, and risk management.
- Scalability and Adaptability: Graph databases handle growing data volumes and complex structures with ease, making them a scalable foundation for AI systems that evolve over time without extensive re-engineering.
- Support for Advanced AI Applications: With the ability to enrich AI models with complex contextual data, graph databases improve model accuracy and support use cases in recommendation engines, generative AI, and anomaly detection.
2. The Long-Term Value of Graph Databases in Enterprise AI
As enterprises look to build more intelligent and adaptive AI systems, graph databases offer a long-term solution that aligns with these goals. Their structure provides a flexible data foundation that can accommodate evolving business needs and data sources, future-proofing AI applications against market changes and technology advancements.
- Resilience and Flexibility: Graph databases allow organizations to adapt quickly to new data types, complex relationships, and increased data volumes. This resilience makes them suitable for AI applications that must scale with an organization’s growth and data complexity.
- Improved Competitive Positioning: Organizations that adopt graph databases as part of their AI strategy can differentiate themselves by delivering highly personalized and responsive customer experiences, optimizing operational efficiency, and reducing risks in real-time. These capabilities are increasingly seen as competitive advantages in sectors like finance, healthcare, and e-commerce.
3. A Call to Action for C-Level Executives
For C-level executives, graph databases represent more than just a data management tool—they are a strategic investment that enables the next generation of AI-driven innovation. As enterprises strive to harness data for competitive advantage, adopting graph databases offers a path to more advanced, intelligent, and adaptable AI applications. Executives should consider several steps to incorporate graph databases into their digital transformation strategy:
- Assess the Current Data Infrastructure: Evaluate whether existing data systems support the complexity and scalability needed for advanced AI applications. If traditional relational databases limit the ability to analyze and leverage interconnected data, a graph database may provide the necessary flexibility and speed.
- Identify Priority Use Cases: Focus on high-impact areas where graph databases can provide immediate value, such as fraud detection, personalized customer experiences, and compliance management.
- Plan for Long-Term Integration: Graph databases are a strategic asset that can grow with the organization. Planning for gradual integration, starting with high-value pilots, can facilitate smoother adoption and scalability.
- Invest in Skill Development: Ensure that data teams are trained in graph database technologies and familiar with graph-specific AI applications. Investing in skill development will maximize the potential of graph databases and support innovative applications.
4. Future Outlook: The Role of Graph Databases in Evolving AI Landscapes
Looking to the future, graph databases are positioned to play a pivotal role in shaping the evolution of enterprise AI. As AI technology advances, enterprises will increasingly rely on graph databases to handle the complexity of data relationships, support new data types, and adapt to the demands of real-time AI applications. Key trends that underscore the importance of graph databases in the future AI landscape include:
- Rise of Generative AI: Graph databases provide the deep contextual data that generative AI models need to produce relevant, accurate, and tailored outputs. As generative AI applications expand, graph databases will be a critical enabler, offering the data depth and relationship insights that drive better AI outputs.
- Growing Demand for Real-Time AI: Real-time AI applications, such as dynamic risk assessment, personalized recommendations, and instant fraud detection, require data systems that support rapid, high-performance querying. Graph databases are well-suited for these applications, as they offer the necessary speed and scalability for real-time processing.
- Increased Focus on Responsible AI and Data Governance: As organizations prioritize responsible AI, graph databases provide the transparency and traceability needed for ethical and compliant AI practices. Data governance and privacy management are integral to modern AI strategies, and graph databases allow organizations to meet these requirements by tracking data lineage and maintaining rigorous access controls.
Graph databases are not only the future of enterprise data management but also a crucial component for unlocking the full potential of AI. By enabling richer data relationships, faster insights, and scalable architectures, graph databases empower enterprises to build more powerful, adaptive, and compliant AI applications. As the AI landscape continues to evolve, organizations that embrace graph databases will be better positioned to innovate, compete, and thrive in a data-driven world.