V. Case Study Applications of Graph Databases in Banking and Finance
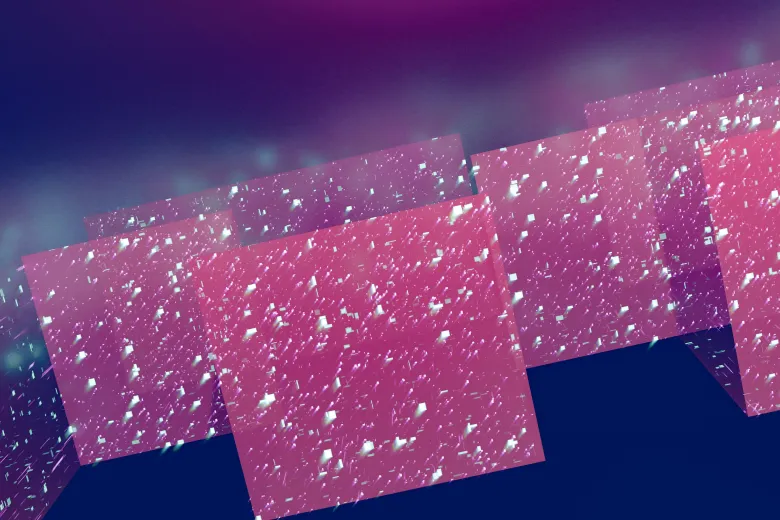
Graph databases are being leveraged by leading financial institutions worldwide to drive significant advancements in areas like fraud detection, personalized customer experiences, compliance, and risk management.
Here are notable case studies demonstrating the transformative impact of graph databases in these areas.
Here are notable case studies demonstrating the transformative impact of graph databases in these areas.
1. Fraud Detection and Prevention: Deutsche Bank
Deutsche Bank implemented graph databases as part of its AI strategy to enhance fraud detection and streamline financial decision-making processes.
The bank used Google Cloud’s generative AI and large language models (LLMs) to derive new insights for financial analysts, improving the efficiency and speed of detecting fraudulent activities. By modeling relationships between transactions, accounts, and associated entities, Deutsche Bank's system can identify suspicious behavior patterns that traditional database structures would miss. This adoption reduced processing time for fraud analysis, making fraud detection faster and more accurate.
https://www.db.com/news/detail/20221207-deutsche-bank-partners-with-nvidia-to-embed-ai-into-financial-services
The bank used Google Cloud’s generative AI and large language models (LLMs) to derive new insights for financial analysts, improving the efficiency and speed of detecting fraudulent activities. By modeling relationships between transactions, accounts, and associated entities, Deutsche Bank's system can identify suspicious behavior patterns that traditional database structures would miss. This adoption reduced processing time for fraud analysis, making fraud detection faster and more accurate.
https://www.db.com/news/detail/20221207-deutsche-bank-partners-with-nvidia-to-embed-ai-into-financial-services
2. Customer Journey Mapping and Personalization: Banco de Crédito del Perú
Banco de Crédito del Perú (BCP) deployed a graph database solution as part of its customer relationship management strategy. This deployment enabled BCP to achieve a 70% increase in insurance sales within the first two months by enhancing cross-selling efforts.
Using AI and graph databases to map relationships across customer data, BCP personalized interactions and targeted customers with relevant offers based on their previous transactions and interactions. The improved understanding of customer journeys also helped the bank refine its overall marketing approach.
https://cloud.google.com/customers/banco-de-credito-del-peru
https://www.mckinsey.com/capabilities/mckinsey-digital/how-we-help-clients/rewired-in-action/bcp-taking-banking-to-new-heights-on-a-digital-rocketship
Using AI and graph databases to map relationships across customer data, BCP personalized interactions and targeted customers with relevant offers based on their previous transactions and interactions. The improved understanding of customer journeys also helped the bank refine its overall marketing approach.
https://cloud.google.com/customers/banco-de-credito-del-peru
https://www.mckinsey.com/capabilities/mckinsey-digital/how-we-help-clients/rewired-in-action/bcp-taking-banking-to-new-heights-on-a-digital-rocketship
3. Risk Assessment: Credit OK
Credit OK, a financial services firm, used graph databases to revamp its credit risk assessment models. This technology allowed the company to analyze complex relationships between applicants, their financial histories, and associated entities to deliver a more nuanced assessment of creditworthiness.
By incorporating graph database insights, Credit OK reported a tenfold increase in deployment speed for its credit risk models, making risk evaluation more efficient and adaptable to high data volumes. This transformation helped Credit OK respond to evolving risk factors and optimize lending processes.
https://cloud.google.com/customers/credit-ok
By incorporating graph database insights, Credit OK reported a tenfold increase in deployment speed for its credit risk models, making risk evaluation more efficient and adaptable to high data volumes. This transformation helped Credit OK respond to evolving risk factors and optimize lending processes.
https://cloud.google.com/customers/credit-ok
4. Compliance and Data Governance: HSBC’s Climate Risk Advisory Tool
HSBC leveraged graph databases within its Dynamic Risk Assessment to manage and assess climate risk factors across its trading portfolios. The system used graph data models to incorporate environmental, social, and governance (ESG) scores from rating agencies, linking these with HSBC’s own trading and asset data.
This setup enabled HSBC to evaluate how climate risk factors could impact the stability and resilience of various portfolios. The tool provided transparent, auditable insights into compliance with evolving regulatory standards, such as climate-related financial disclosures, while helping HSBC align with sustainability initiatives.
https://www.hsbc.com/-/files/hsbc/investors/hsbc-results/2022/annual/pdfs/hsbc-uk-bank-plc/230221-annual-report-and-accounts-2022.pdf
Recommendation Engines for Financial Products: ING Bank
ING Bank incorporated graph databases to improve its chatbot and recommendation systems, focusing on personalized customer interactions. By integrating Vertex AI for natural language understanding and graph-based data, ING's chatbot could identify customer needs in real-time and suggest relevant financial products or services. This AI-driven, graph-based setup provided a 30% reduction in operational costs while improving customer satisfaction, as the chatbot could access connected data to deliver accurate, context-aware responses.
These real-world case studies demonstrate the substantial value that graph databases bring to AI applications in the banking and finance sector. From improving fraud detection speed and precision to enhancing personalization in customer service, graph databases support more efficient, scalable, and adaptable AI models. Financial institutions adopting these solutions are better equipped to navigate the complex landscape of regulatory compliance, customer expectations, and operational risks. These case studies underscore the role of graph databases as a crucial enabler for advanced AI applications, setting a foundation for continuous innovation in data-driven financial services.
https://www.ing.com/Newsroom/News/ING-selected-as-one-of-worlds-four-most-innovative-banks.htm
This setup enabled HSBC to evaluate how climate risk factors could impact the stability and resilience of various portfolios. The tool provided transparent, auditable insights into compliance with evolving regulatory standards, such as climate-related financial disclosures, while helping HSBC align with sustainability initiatives.
https://www.hsbc.com/-/files/hsbc/investors/hsbc-results/2022/annual/pdfs/hsbc-uk-bank-plc/230221-annual-report-and-accounts-2022.pdf
Recommendation Engines for Financial Products: ING Bank
ING Bank incorporated graph databases to improve its chatbot and recommendation systems, focusing on personalized customer interactions. By integrating Vertex AI for natural language understanding and graph-based data, ING's chatbot could identify customer needs in real-time and suggest relevant financial products or services. This AI-driven, graph-based setup provided a 30% reduction in operational costs while improving customer satisfaction, as the chatbot could access connected data to deliver accurate, context-aware responses.
These real-world case studies demonstrate the substantial value that graph databases bring to AI applications in the banking and finance sector. From improving fraud detection speed and precision to enhancing personalization in customer service, graph databases support more efficient, scalable, and adaptable AI models. Financial institutions adopting these solutions are better equipped to navigate the complex landscape of regulatory compliance, customer expectations, and operational risks. These case studies underscore the role of graph databases as a crucial enabler for advanced AI applications, setting a foundation for continuous innovation in data-driven financial services.
https://www.ing.com/Newsroom/News/ING-selected-as-one-of-worlds-four-most-innovative-banks.htm